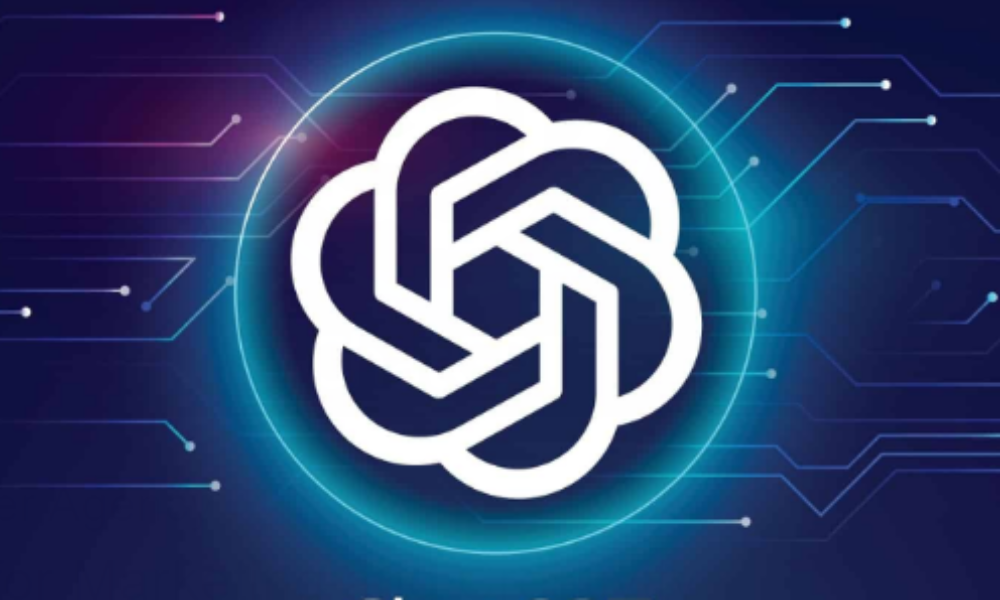
More Human Than Human?
How ChatGPT is Mirroring Biases in Humanity
R. Jiang
“DELVE” AND “TAPESTRY” HAVE BEEN RUINED FOREVER. Nothing screams ChatGPT more than delving into rich tapestries. But why is this the case and where did this come from? The graph below speaks for itself. We cannot deny the extent to which generative AI models have influenced the way we write, and by extension, talk. Therefore, as the application of large language models (LLMs) grows and broadens in its scope and reach, we must be critical of their output. One must realise that large language models are inherently biased.
What Actually Are LLMs?
LLMs are systems that are used to model and process human language. It is a type of AI algorithm that teaches computers to process data in a way that is inspired by the human brain in order to summarise, generate, and predict new content. The reason why they are ‘large’ is because the model often requires millions or even billions of text data, which is used to train the model and teach it what to say and when. This method of training using a sample or set of data is where the problem begins.
More Than One Type Of Bias?
According to Forbes, there could be as many as five different types of biases in ChatGPT. One type of bias is pretty self-evident, and is inherently obvious. After all, only a subset of the entire English language has been used to train LLMs.
The diversity of the data – or lack thereof – will almost certainly have an impact on the output of an AI, although this issue has been slightly remediated with the introduction of internet access. This is called selection bias. This happens when the data is not representative of what it is meant to model. Incomplete data or biased sampling may be some factors that lead to a biased training dataset.
Therefore, the nature of LLMs means that their performance is limited to the actual dataset used to train such models.
Human Biases?
Since these generative AI models aim to possess a high understanding of the human language and its patterns, they are able to produce natural and human conversations. However, the ultimate limitation is that our existing prejudices are being exacerbated by such models due to the fact that data sets will always be biased. So maybe it is not the model that produces these biases, but rather magnifies the effect of such. Examples include political bias or discriminatory prejudices.
Perhaps ChatGPT will always be biased, however such an innovation has allowed us to realise that cognitive biases are everywhere, and will always be a defining element of humanity.